Psychophysiological Measures of Workload During Continuous Manual Performance
Changing work conditions, physiological measurement possibilities, and possible statistical consequences
Competitive pressure, digitization, and constant technological progress are contributing to the increasing informational load of work processes, especially in areas where formerly standardized and routine-heavy processes were dominant (e.g. manual assembly). These changing work conditions do not only impact the individual worker's (mental) workload but also necessary ergonomic countermeasures. A clear tendency away from the classical biomechanically influenced ergonomic view towards a more mentally and information processing focused neuroergonomic perspective is already evident. This trend is supported by advancements in the area of psycho- and neurophysiological measurement equipment. It becomes more mobile, cheaper due to an increasing customer market, more precise (e.g. through increasing sampling frequency or better temporal or spatial resolutions), and thus overall more applicable at the concrete workplace and not only in laboratory settings.
Using physiological measurements to derive ergonomic countermeasures from these data requires special statistical methods. Following the established methods of mean comparisons over longer lasting time frames and/or groups of workers undermines the opportunities temporally high resulted data offers. The final aim using such data should be to objectively assess mental workload (MWL) outside the lab at real work places and to improve (or restructure) work processes in a way that keeps workers in a productive and efficient range of MWL.
Taking neurological methods as a model, this paper will discuss new approaches to analyze physiological data during work processes, highlighting the chances of increasingly finer differentiation and temporal resolution. While those methods are already common for EEG, fMRT, or fNIRS data, ECG, GSR and Eye Tracking data are still mainly processed in a different way. Several analysis approaches to analyze individual as well as group data will be discussed. Differences between event-related and block (or work process) based mean comparison approaches will be shown as well as the usage of event-related pattern analysis (on an individual as well as group level). Finally, a theoretical framework for pattern-based analysis of physiological data will be presented coupling theoretical assumptions and machine learning possibilities. Measuring, documenting, and analyzing physiological based MWL data during work processes can help to keep workers healthy for a longer period of time, and will also provide the basis for workload adaptive (informational) work assistance systems.
Measuring mental workload at the work place
Physiological states constantly undergo fluctuational changes. Some changes occur via natural organismic processes (breathing, heat compensation), while others are the result of confrontations with changing or unexpected (work) stimuli. In ergonomic research, such cue- or event-related arousal changes are of great interest, as these changes are seen as indicators of increasing or decreasing workload. Using physiological measurement techniques, a non-interruptive observation of these (mental or physical) load patterns becomes possible.
Defining and operationalizing mental workload
While there was and is little dissensus in ergonomics that the study of MWL is fundamental to the understanding of functions and limitations of the human information processing system (Wickens 2017), measurement and analysis strategies as well as operationalization of MWL remain highly discussed topics (Van Acker et al. 2018; Dehais et al. 2020). MWL has a long history in human factors research. Physiological activation was connected to the prediction of task performance. Later the concept of this relationship was extended, associating it with the idea of a finite information processing capacity which is confronted with variable cognitive demands. Thus, the first central proposition postulates a fit or a misfit between external demands and internal capacitively limited resources. The second proposition concerns the problem of an occurring misfit and if there is a possibility to dynamically cope with it in order to compensate and avoid states of longer lasting (hypo- or hyper‑) stress and discomfort (Selye 1974). Both propositions are the basis of a dynamic adaptive approach, which assumes that in general, but especially in the case of misfit, the organism is seeking for a balanced state of homeostasis and cognitive comfort (Hancock and Warm 1989; Dehais et al. 2020). Finally, there is the assumption that misfits of longer duration should be ergonomically countermeasured.
While these basic assumptions are commonly shared between most researchers, the topics of concrete operationalization and measurement are widely discussed and applied methodologies vary greatly. Different approaches exist to measure MWL using either subjective ratings, more objective performance or observational data, or measuring a wide range of neuro- and psychophysiological indicators. All those methods have in common that they assume to be able to record the individual workers changes of MWL at the work place. A wide range of studies was conducted proving in either laboratory or field settings that those indicators are able to differentiate between levels of MWL (Myrtek et al. 1994; Marquart et al. 2015; Delliaux et al. 2019; Jafari et al. 2020), assembly products of different complexity (Bläsing and Bornewasser 2020, 2021), or even to detect potential mental overload (Hoover et al. 2012). Regarding the measurement of MWL the most discussable points seem to be which indicator to choose, how to analyze it, and if all those indicators are measuring the same aspect of MWL or if it is a rather multidimensional construct (Matthews et al. 2015a).
To a large extent, measuring MWL is a rather practical problem. The operationalization of MWL, on the other hand, is a more theoretical concern. While the concept of the existence of a limited (cognitive) resource that is necessary to cope with external demands is intuitive and easy to understand, theories tend to remain on a rather abstract and descriptive level. Using neurophysiological measurements, it becomes possible to begin to better understand the basic processes of attention and behavior (Parasuraman 2011), but a unified theory of MWL, that merges theoretical aspects from neuroscience, human factors and ergonomics, as well as basic physiology is still missing (Dehais et al. 2020).
Sufficient digital models for mental workload (MWL) and adaptational processes (especially concerning states of over- and underload) do not yet exist. Digital process and strain models probably offer a better chance for a meaningful integration of the measured parameters into some kind of a cognitive overload risk analysis. In a more ergonomical direction, an indicator of this kind could even become the basis for automated workload-matched adaptations for e.g. information assistance systems. However, this approach implies that we are able to measure workload in real time, that we can assess the real amount of event related workload in absolute values, and that we can define redlines, which dynamically separate e.g. regions of reserve capacity and regions of overload from a region of comfort (Young et al. 2015). Until now, none of these implications is completely fulfilled.
Approaches to mental workload measurement
MWL arises in the area of tension between task-related requirements and personal resources, experiences, and competencies, and thus represents a dynamic and highly interindividual different phenomenon. Being able to validly assess MWL at the work place has to become the basis of ergonomical countermeasures. Thus, a deeper consideration of the different measurement possibilities (subjective, performance related, observational, and physiological; Chen et al. 2016; Longo 2018) becomes necessary. Most use cases and laboratory settings are using a combination of different indicators to compensate for their individual weaknesses and combine their strengths. The combination of physiological measurements and observational data enables to control the analysis for non-work-related distractions or focus on concrete event-related changes. However, psychometric analysis casts doubt on the assumption that the combination of different MWL indicators might always be sufficient. Matthews et al. (2015b) showed that single indicator approaches were sensitive but occasionally contradictory. To avoid such dissociative results, the used approaches have to be precisely coordinated.
Subjective ratings and similar methods might not be suited for just-in-time detection of MWL changes. Automatically (mechanically) recorded performance parameters, as well as observational data (e.g. in the form of timestamps from machines and assistance systems) have to be focused and combined with objectively measurable physiological changes to fully cover work process related MWL changes.
Improved and miniaturized sensor technologies, resulting in the possibility to most widely measure continuous, mobile, and non-interruptive directly at the work place, have led to an increase in reception for such measurements in recent years (Charles and Nixon 2019). Products originally coming from the consumer market have received increasing interest in human factors and ergonomics research, and have accelerated technological improvement even for more professional equipment. As a consequence, mobile devices are not only increasing in resolution (resp. sampling frequency), but also decreasing in acquisitional costs. At the same time, individuals become more used to wearing them and open-minded to quantify themselves (Swan 2012). These effects pave the way for a broader use in field research. Where procedures such as electrocardiography (ECG) (Mulder 1992; Sammito et al. 2015) and the measurement of muscle activity (EMG) (Kluth et al. 2013) have been already widely used, entirely new possibilities are now opening up, e.g., via mobile eye-tracking and mobile electroencephalography (EEG) solutions (Wascher et al. 2020), allowing for broader objective insights into the processes of workers MWL.
The basic assumption of those rather psychophysiological measurement methods is that the activation of resources to cope with changing work stimuli leads to a measurable change in the activity of the autonomous nervous system (ANS) (Oken et al. 2006; McEwen and Gianaros 2011; Jarczok et al. 2013). While this can be either seen as a more homeostatic process to keep the individuum in a balanced state (Ramsay and Woods 2014), or just a reaction of the bottlenecked information processing in the working memory (Baddeley 2003; Chen et al. 2016), the change of the measured indicators is always interpreted as a momentarily, simultaneously occurring change in MWL.
Neuroergonomics focus more on the usage of modern imaging techniques like functional infrared spectroscopy (fNIRS) or functional magnet resonance imaging (fMRI). Those techniques enable a deeper understanding of concrete neurophysiological brain reactions to concrete work stimuli using an improved spatial and temporal resolution. The idea of resources as the main point for MWL is displaced in favor of an idea of concrete neurophysiological markers and degraded mental states. MWL becomes a measurable brain state. These techniques represent an attempt to open the former black box of MWL and to predict which neuronal and metabolic states lead to decreased performance.
This paradigm shift contrasts classical psychophysiological approaches (Backs and Boucsein 2000), where researchers are rather focused on peripheral correlates of unknown central, neuronal, or metabolic processes. Of course, brain activity and heart rate both are not identical to MWL, but where a neuroergonomist believes he is already inside or closest to the brain and can directly observe brain functions, a psychophysiologist still believes he is outside but has a better chance to take a rough look on information processes and MWL. Inherently, both perspectives assume to validly measure the true amount of MWL.
In this article the focus will be on some of the most widely used (and easiest to apply) psychophysiological measurements. Using heart rate (HR) and different heart rate variability (HRV) indicators as ECG derivates, it becomes possible to relate changing MWL and changing cardiovascular activity to each other. The used HRV indicators are mostly time-based which, due to their mathematical basis, makes them easier to calculate and assess in a dynamic, and, perceptively, just-in-time manner. HR and HRV have already been proven to be able to differentiate between levels of MWL (Myrtek et al. 1994; Delliaux et al. 2019), even using first machine learning approaches to quantify those changes (Hoover et al. 2012). Furthermore, gaze and pupil related parameters like pupillary response (PR), fixation duration (FD), or saccadic peak velocity (SPV) derived from eye tracking measurement are able to show physiological changes with a direct relationship to information processing resp. changing informational load (Di Stasi et al. 2010; Marquart et al. 2015; Di Nocera et al. 2016; Herten et al. 2017; Mathôt 2018).
Although there are different positions, research agrees that it is possible to detect meaningful changes of MWL during work processes using either neuro- or psychophysiological measurement techniques. To interpret changes e.g. of heart rate as changes in MWL, the high complexity and the dynamics of MWL distribution in the working process need to be assessed (exemplarily in Bläsing and Bornewasser 2020). During working processes under real life conditions, the amount of information to be processed is constantly changing and so is the resulting MWL. Thus, continuously changing MWL becomes an elementary part of each working process, fluctuating in dependent on the current informational load. For a better understanding of such processes, it is necessary to develop an analysis framework, which not only takes these special conditions into account, but even actively highlights them.
Analyzing mental workload
Traditionally, MWL research focuses on longer analysis durations to investigate effects of work on an individuals perceived work load. For example, complete assembly processes or sections lasting several minutes are compared with each other or are used to create progress profiles (Nardolillo et al. 2017; Bläsing and Bornewasser 2021). The advantage of such an approach is that for analysis, mostly aggregated and cleaned data can be used, which are less susceptible to measurement errors, spontaneous and non-task related variations, and general noise. Stress periods of short duration are neglected, as are continuous, slow increases, which might indicate increasing fatigue (Marandi et al. 2018). As a result, analysis protocols are produced that show the distribution of MWL aggregated over different subjects and over sections of different time lengths.
Multimodal physiological MWL measurement—timeframe and analysis level dependency
Physiological indicators of MWL vary in their respective latency until changing conditions will be observable. While HR changes rather slowly with a latency up to 30 s (comprising multiple cardiac cycles), PR changes in parts of seconds. Using a multimodal measurement approach might therefore help to identify different aspects of MWL. But alone the choice of a multimodal approach will not automatically help to get the most information from a given situation. Using the standard analysis strategy of mean value comparison over longer periods might neglect the influence of short durations of higher MWL. But those time points are the most valuable ones from an ergonomic point of view, because they offer the chance for adaptation and improvement. Readjusting the timeframe by increasing the timewise granularity (shorter durations) to be analyzed can be a first step to get a better insight into work process related effects on workers MWL.
Table 1 reports mean values and standard deviations for various psychophysiological indicators with a close relation to MWL. Data was gathered during an experiment where participants had to assemble a miniaturized truck support framework (Bläsing and Bornewasser 2020). The assembly instruction consisted of three steps, during which participants had only a limited time frame to memorize all necessary information, thus leading to an increase of MWL. The presented data are aggregated from 39 participants. The total assembly value represents the mean value during the whole assembly process, neglecting individual differences in execution and speed. Step 1 to step 3 where standardized with 20 s analysis intervals. Taking a closer look at these data, some differences can be shown (e.g. HRV indicators rrHRV and SDNN or eye-tracking and gaze behavior related fixation duration and saccadic peak velocity). However, with this procedure, it is still not possible to draw conclusions about the distribution of possible short peaks or longer lasting changes (towards more or less MWL). In addition, it can be seen that standard deviation for all parameters was higher during the shorter analysis periods, thus indicating higher interindividual differences. Analysis of MWL therefore becomes not only dependent on the used time duration, but also of the analysis level—group or individuum based.
Fig. 1 shows the occurring fluctuations of different psychophysiological parameters on an individual level. It is possible to see the ECG derivates HR (solid line) and rrHRV (dotted line), as well as PR, and the gaze related parameters FD (solid line) and SPV (dotted line) during the assembly of one truck support framework. Black vertical lines indicate interactions with an assembly assistance system, which provided new assembly instructions. Clear fluctuations can be spotted for all parameters with for example beats per minute varying between 70 and 87. A closer look at the HR trajectory reveals that there is not a clear increasing and declining tendency, but various short peaks of different length and height, even though the total assembly duration is only seven minutes.
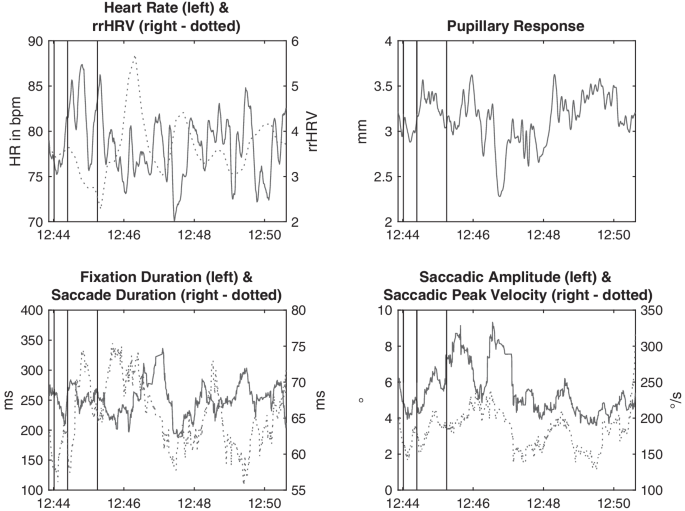
Trajectories of physiologically based mental workload parameters during the manual assembly of a truck support framework
Schwankungen physiologischer Indikatoren mentaler Beanspruchung während der manuellen Montage eines LKW-Hilfsrahmens
Increasing timewise granularity (zooming in) while being able to perform analysis on a group level requires accurate time stamps for points of interest during the measurement. Compared to a standardized laboratory task, where the analysis time can be adjusted to the duration of the stimulus presentation, field measurements require the precise acquisition of timestamps for each participant. Taking an assembly task for an example, dependent on prior knowledge, individual competencies, and work strategies, participants will reach the same assembly step at different times. To analyze and compare the data of this assembly step over all participants, a (preferably automated) timestamp is required. But even with an automated timestamp, not only interindividual differences in competencies will lead to different levels of MWL, also different physiological prerequisites will lead to different (possible) reactions. Taking HR and HRV as examples, they are both highly dependent on numerous factors like fitness, age, and substance consumption (Sammito and Böckelmann 2016) and thus even the physiologically possible variance (the steepness of an in- or decrease) differs between them. Additional improvements have to be made to boost the amount of information one can get from the data.
Event vs. block analysis
Neurological research differentiates the analysis and study design for physiological measurement series (e.g. fMRI studies) in either event or block-based designs (Buckner et al. 1996). Both analysis paths are able to be performed on an individual or groupwise base. The main differences are in the phenomena to be captured (time-sensitive or not-time-sensitive) and the controllability of the participant. All studies where the participant is able to trigger the response (participant is in full control of the situation) are event-based, while a design where all stimuli are controlled from the study itself is block-based. Applying this differentiation to a field resp. work setting, event-based might be a rather unstandardized assembly process at a mixed model assembly station with higher degrees of freedom for the worker vs. clocked assembly line work where the work process defines at what time the worker needs to interact with a new stimulus (block-based).
From an ergonomic and MWL point of view, the chance that event-based scenarios will lead to an increase of MWL are enhanced due to their higher unpredictability and higher need of adaptation. In such unstandardized situations, more information needs to be processed and neither skill nor rule based behavior can be shown (Rasmussen 1983). Advantages of a block-based design with externally specified timeframes lie in eased group comparisons and increased stability against artefact, noise, and shorter unimportant physiological changes.
Fig. 2 illustrates an individual HR course over five minutes. Using a block analysis strategy, mean HR would be 76.66 and rrHRV 3.87. During this five-minute section, two peaks with HR levels over 90 beats per minute (bpm) can be seen, as well as a short period below 70 bpm. Using a block design with a 300 s duration would ignore both phenomena, potentially leaving at least two process steps left that could have been optimized. Conversely, an event-based approach might be able to identify those short increases as well as the phase below the mean, if a tracked event took place around this time.
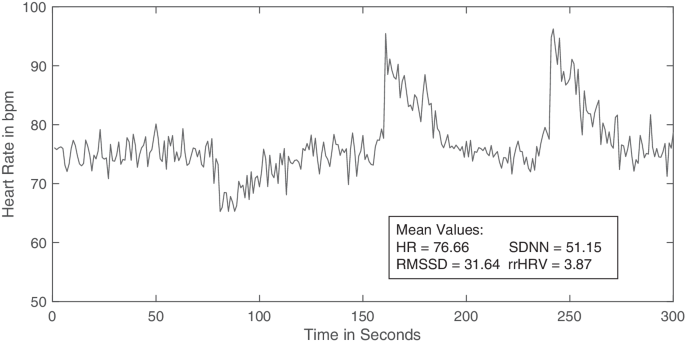
Differences between block and event design using the example of HR
Event- und Block Design im Vergleich am Beispiel der Herzfrequenz
Multidimensional approach using an event-based group comparison
Most work processes have at least some repetitive aspects. Searching assembly parts, screening a monitor, or interacting with a user interface can be seen as such a repetitive task that should always create similar MWL reactions, and thus physiological response pattern. If there is a clear, distinguishable, and time-wise delimitable event, event-related responses can be further analyzed by using selective averaging either on an individual or group level. Fig. 3 illustrates such an approach for several similar events for one person and the resp. HR and PR pattern. The solid dark-grey lines indicate the moving averages over all events and show clearly visible patterns, indicating an increase in arousal (or MWL) shortly after (HR) or before (PR) the event (solid vertical black line) took place. To increase compatibility and ease the interpretability, as well as the possibility to use this method for a groupwise comparison, all data in the one-minute time window where min-max-transformed to offer the chance to indicate (and calculate as well) local max- and minima. For statistical analysis, repeated measures ANOVAs could be used to identify differences pre-, during, and post the relevant event.
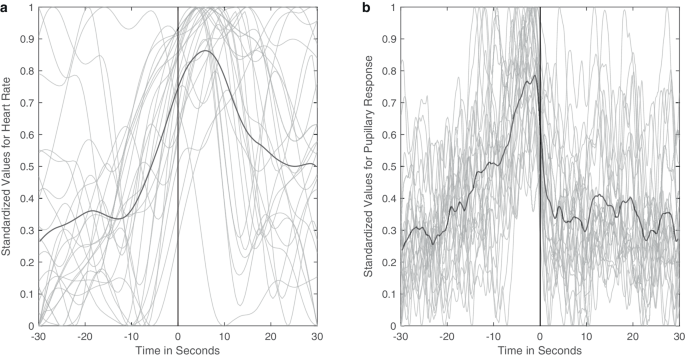
Selective averaging for event-related responses (HRa, PRb)
Selektive Mittelwertbildung für eventbasierte Reaktionsmuster (Herzfrequenza, Pupillenausdehnungb)
The similarity of the events used in Fig. 3 was high enough to result in nearly identical reaction patterns. Some shifts along the time-axis occurred mainly due to unprecise event time stamp recording or irregularly occurring measurement disturbances. Fig. 4 highlights the importance of such a similarity for a meaningful interpretation of the results. Solid dark-grey lines show the average means over all three interactions with the assembly system during the assembly of six truck support frameworks of different complexity (overall n = 702). For the dark-grey lines, only changes for FD and PR are clearly apparent, the remaining physiological parameters are only meandering around the overall mean. Refocusing on the different assembly steps revealed clearly distinguishable patterns for most indicators (grey lines in the background). Taking a closer look at the three assembly steps, differences in informational load of each step could have been identified, leading to different MWL reaction patterns (Bläsing and Bornewasser 2020).
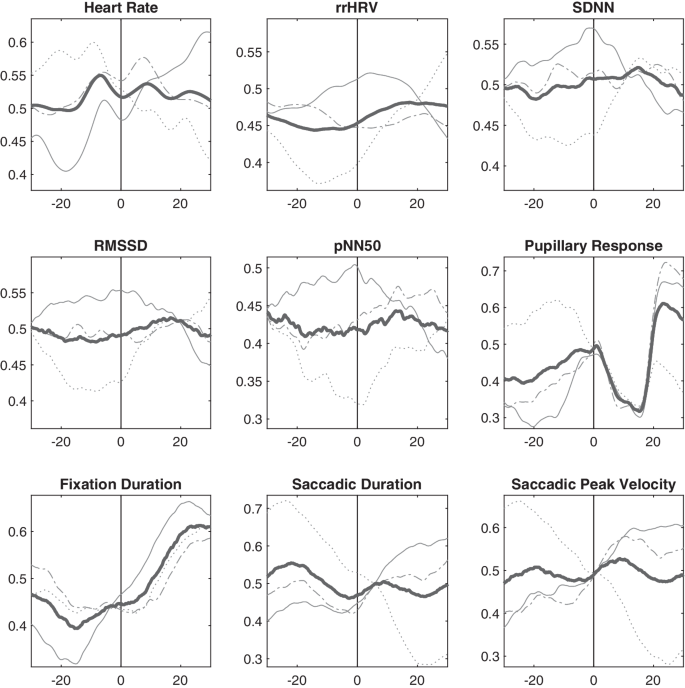
Standardized physiological reaction patterns during first-time confrontation with new stimuli (vertical-black line) during manual assembly of a truck support framework (dark-grey = mean over all assembly steps, light-grey-solid = step 1, light-grey-dotted = step 2, light-grey-dots-and-lines = step 3). (For more information see Bläsing and Bornewasser 2020)
Standardisierte physiologische Reaktionsmuster während der erstmaligen Konfrontation mit neuen Stimuli (schwarze-vertikale Linie) im Rahmen der manuellen Montage eines LKW-Hilfsrahmens (grau = Mittelwert über alle Montageschritte, hellgrau = Schritt 1, hellgrau (Punkte) = Schritt 2, hellgrau (Punkte und Striche) = Schritt 3). (Mehr Informationen in Bläsing and Bornewasser 2020)
In summary, multimodal physiological measurements are a useful approach to gain insight into an individual's MWL. When using such methods, it is necessary to decide which analysis strategy fits the given situation best. Laboratory approaches cannot be adopted to field settings one-to-one. The described methods all have in common that they are highly dependent on exactly measured time-stamps and that the work events of interest are already known. Block-wise analysis approaches can be used meaningfully if concrete processes (of equal length if possible) have to be compared, while event-based analysis can help to identify concrete problematic process parts and design ergonomic countermeasures. For a comprehensive (cognitive) risk analysis, a mixture of both approaches becomes the best solution. While block-wise approaches are able to identify critical processes based on the experience of multiple workers, event-based approaches focus more on case-analysis and the individual's experience.
Real-time workload classification using peaks and plateaus as an alternative analysis approach
The approaches described above primarily focus on the usage and availability of event-related timestamps. Today, such timestamps are often missing or only available with lower temporal resolution (e.g. on the level of entire assembly models, but not on the level of process steps). Eye tracking and gaze behavioral data can be used as bridging technologies to post-hoc estimate the interaction time, but on the long run, data from linked machines (Internet of Things) are necessary. Connecting those automatically generated timestamps with relevant physiological data enables the identification of ANS based arousal changes, which are interpreted as changes in MWL. Thus, additional analysis strategies have to be considered to validly connect the ups and downs of MWL amidst occurring changes in the work process.
Of peaks, plateaus, and noise
Missing timestamps therefore complicate the interpretation of physiological reaction patterns. Coming from the field of signal detection theory, a continuous data flow might include relevant signal parts (e.g. reactions to concrete events) and additional noise. In terms of physiological measurements and MWL, noise would indicate phases without significant changes, mainly characterized by naturally occurring fluctuations and minimal changes in a corridor one might describe as the same workload level. Classical analysis techniques with fixed analysis durations include a lot of noise and therefore highlight MWL on a long-term and surface level. The following framework is based on three typical occurring phenomena or reaction patterns during physiological data assessment: undefined/noise, short peaks, and longer lasting plateaus. It is assumed that each course of MWL can be either classified as noise or as an interplay of peaks and plateau phases.
Fig. 5 visualizes all three possible outcomes. Noise characterizes parts with no additional information. The duration (d) of those parts is without interest, as long as the total amplitudal changes (a) during this period stays in a predefined range. If an amplitudal change greater than the defined threshold is detected within a noisy part, it has to be either a peak or a plateau. The excess of the threshold adds information to the former part of noise. Due to the significant measured change, the noisy part can become a plateau, and serve as a new baseline. Thus, noise can be seen as some kind of plateau without added informational value. Without at least a peak or another plateau, it is not possible to characterize the MWL during a noisy phase. It can only be stated, that MWL underwent no significant changes during this period.
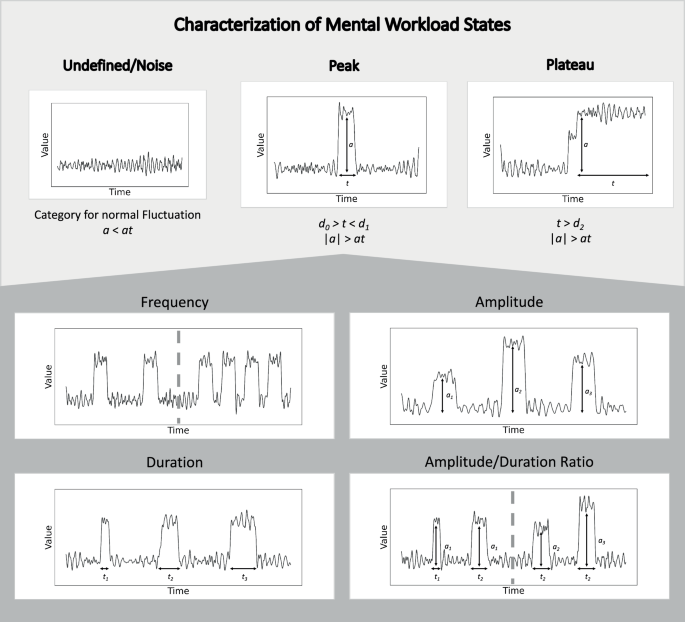
Characterization of MWL states (d 1,2 = predefined time values, t = duration, a = amplitude, at = predefined amplitudal threshold). Trajectories are generated using the Python NeurKit2 toolbox. (Makowski et al. 2021)
Charakterisierung potenzieller MWL Zustände (d 1,2 = vordefinierte Zeitwerte, t = Dauer, a = Amplitude, at = vordefinierter Amplitudenschwellwert). Die Verläufe wurden mit der auf Python basierten NeurKit2 Toolbox erstellt. (Makowski et al. 2021)
Conversely, peaks and plateaus contain informational value. Both indicate MWL changes over a specific time. A peak is characterized by a comparatively short time span (t < d1) and a high amplitude (a) with even abruptly occurring changes. In- and decrease have to appear in a given timeframe (d1). An exception can be a peak at the end of a measurement period, where the decrease might not be a necessary prerequisite. A peak has not necessarily to be an amplitudal change in a positive direction, decreases (drops) exceeding the same amplitudal threshold (|a| > at) can also be classified as peaks. Amplitude-wise plateaus have similar prerequisites. The main difference is their temporal extension.
Peaks, plateaus, and noise arise through the interaction of an individual worker with steadily changing work conditions and can be used to better quantify this highly interactive process between internal resources and external demands. Results of those analysis are still dependent on some settings. A plateau can contain some peaks, and a different granularity level might reveal plateaus where before just noise was detectable. In addition, manipulating amplitude threshold (at) and duration values will change the results. Necessary characterization values for peaks and plateau detection (duration and amplitudal threshold) are i.a. dependent on the chosen parameter's latency (the time until changes are noticeable), adaptability, and possible range of measurable differences.
Detailed peak and plateau analysis: frequency, amplitude, and duration
If peak and plateau detection is automatized, the characterization of those phenomena can add additional information to the analysis and even have implications for ergonomical countermeasures. Peaks and Plateaus may vary in their number of occurrences in a given time (frequency), their total amplitude, duration, or the ratio of amplitude and duration (see bottom part of Fig. 5). All those analysis strategies should improve the understanding of MWL changes in a process orientated approach. Those characterizations can be used to compare different work processes or even workers. The appearance of high frequent peaks, peaks of higher amplitude, or especially longer plateaus of higher MWL can indicate that the process needs improvement or the worker needs support.
Pattern analysis and machine learning
We are living in the age of data with large amounts of data gathered every day. Especially when using physiological measurement devices with continuous data streams, the amount of information becomes too large to manually handle. Methods from data science, machine learning, and artificial intelligence can and should be used to get the most out of the gathered data. Increased processing power, improved algorithms, and mobile data collection will enable the automatized and MWL based control of, for example, adaptive informational assistance systems (Bläsing and Bornewasser 2019). First promising approaches using HR to (live) detect MWL changes have existed for years (Hoover et al. 2012), so in the near future, a better understanding of work processes and live adjustments on the job will be possible based on individual MWL data.
When using machine learning in MWL research and practical application, the full range of available algorithm classes will be needed. With the main aim of either detecting deviating behavior or classifying momentary physiological patterns as a specific subset of MWL, unsupervised as well as supervised algorithms are needed. To identify more complex relationships between physiological and machine data deep learning approaches might help. Yet, it has to be stated that not all algorithms can cope with physiological data, especially with the problem of simultaneously measured channels of different devices (e.g. ECG, eye tracking, EEG) and their meaningful interaction (Barua et al. 2015, 2020). Furthermore, the varied latencies of the used parameters complicate the application. Future research is necessary to overcome these limitations and enable an appropriate use and meaningful interpretation of machine learning in MWL research and practice.
Conclusion
The strategies and framework described above represent attempts to connect theoretical MWL constructs with a variety of physiological indicators to make MWL a more tangible phenomenon. For a better understanding, and to be able to react in an ergonomical meaningful way, an interpretation of individual physiological trajectories is only possible amid the presence of a concrete event. Events can either be analyzed using predefined timestamps or using modern machine learning and data science approaches to post-hoc connect prominent reaction patterns with machine data.
Within the present framework, several research questions can be derived. For example, future research might investigate what patterns of psychophysiological reactions indicate MWL stability and which should be stated as remarkable changes. In addition, borders of MWL (like Young et al. (2015) propose them), as well as the number of different MWL levels should be discussed. Furthermore, the topic of distinguishability between physical, mental, and even emotional load at the work place should be addressed. Especially physical load can lead to masking effects using HR or HRV as indicators of MWL, due to the bigger impacts of physical work on the cardiovascular system (Backs et al. 1994). A combination of different indicators might represent a solution, as long as these indicators can be connected in a consistent and theoretically derived way.
While the combination of different physiological parameters to one MWL indicator offers some advantages, it is still unclear if different parameters, for example HR and PR, might only be representative for special subtasks during human information processing. Accordingly, changes in HR and PR might only correlate with those tasks. Sensory and decision-making processes might be linked to different indicators and might be represented by different patterns in duration and amplitude. Machine learning approaches can help to combine the information from different indicators, without losing the information of single indicators.
Using in-depth analysis of physiological data to classify MWL (and MWL changes), there seems to be a fine line between differences that are detectible and differences that are significant in content. A statistically significant difference does not necessarily lead to meaningful conclusions, as well as the absence of such differences does allow the conclusion that the work process is not ergonomically improvable. The final decision should always be left to a human being. Machine learning and pattern analysis should be seen as useful but fallible tools.
Even with theoretical hurdles still existing and challenges that need to be overcome, especially concerning alignment of multimodal data, movement artefacts, and further measurement deficiencies, in-depth analysis of continuous physiological data might be an important intermediate step between the state of the art in ergonomic research and the prospective application of machine learning algorithms to automatically and just-in-time classify MWL.
References
-
Backs RW, Boucsein W (2000) Engineering psychophysiology: issues and applications. Lawrence Erlbaum, Mahwah
-
Backs RW, Ryan AM, Wilson GF (1994) Psychophysiological measures of workload during continuous manual performance. Hum Factors 36:514–531
-
Baddeley A (2003) Working memory: looking back and looking forward. Nat Rev Neurosci 4:829–839
-
Barua S, Begum S, Ahmed MU (2015) Supervised machine learning algorithms to diagnose stress for vehicle drivers based on physiological sensor signals. Stud Health Technol Inform 211:241–248
-
Barua S, Ahmed MU, Begum S (2020) Towards intelligent data analytics: a case study in driver cognitive load classification. Brain Sci 10:19
-
Bläsing D, Bornewasser M (2019) A strain based model for adaptive regulation of cognitive assistance systems—theoretical framework and practical limitations. In: Karwowski W, Ahram T (eds) Intelligent human systems integration 2019. Springer, Cham, pp 10–16
-
Bläsing D, Bornewasser M (2020) Influence of complexity and noise on mental workload during a manual assembly task. In: Longo L, Leva MC (eds) Human mental workload: models and applications. Communications in computer and information science. Springer, Cham, pp 147–174
-
Bläsing D, Bornewasser M (2021) Influence of increasing task complexity and use of informational assistance systems on mental workload. Brain Sci 11:102
-
Buckner RL, Bandettini PA, O'Craven KM, Savoy RL, Petersen SE, Raichle ME, Rosen BR (1996) Detection of cortical activation during averaged single trials of a cognitive task using functional magnetic resonance imaging. Proc Natl Acad Sci 93:14878–14883
-
Charles RL, Nixon J (2019) Measuring mental workload using physiological measures: a systematic review. Appl Ergon 74:221–232
-
Chen F, Zhou J, Wang Y, Yu K, Arshad SZ, Khawaji A, Conway D (2016) Robust multimodal cognitive load measurement. Springer, Cham
-
Dehais F, Lafont A, Roy R, Fairclough S (2020) A neuroergonomics approach to mental workload, engagement and human performance. Front Neurosci 14:268
-
Delliaux S, Delaforge A, Deharo J‑C, Chaumet G (2019) Mental workload alters heart rate variability, lowering non-linear dynamics. Front Physiol 10:565
-
Di Nocera F, Mastrangelo S, Colonna SP, Steinhage A, Baldauf M, Kataria A (2016) Mental workload assessment using eye-tracking glasses in a simulated maritime scenario. In: Proceedings of the human factors and ergonomics society europe chapter 2015 annual conference, p 14
-
Di Stasi LL, Renner R, Staehr P, Helmert JR, Velichkovsky BM, Cañas JJ, Catena A, Pannasch S (2010) Saccadic peak velocity sensitivity to variations in mental workload. Aviat Space Environ Med 81:413–417
-
Hancock PA (1989) A dynamic model of stress and sustained attention. Human Factors 31(5):519–537. https://doi.org/10.1177/001872088903100503
-
Herten N, Otto T, Wolf OT (2017) The role of eye fixation in memory enhancement under stress—An eye tracking study. Neurobiol Learn Mem 140:134–144
-
Hoover A, Singh A, Fishel-Brown S, Muth E (2012) Real-time detection of workload changes using heart rate variability. Biomed Signal Process Control 7:333–341
-
Jafari M, Zaeri F, Jafari AH, Payandeh Najafabadi AT, Al-Qaisi S, Hassanzadeh-Rangi N (2020) Assessment and monitoring of mental workload in subway train operations using physiological, subjective, and performance measures. Hum Factors Ergon Manuf Serv Ind 30:165–175
-
Jarczok MN, Jarczok M, Mauss D, Koenig J, Li J, Herr RM, Thayer JF (2013) Autonomic nervous system activity and workplace stressors—A systematic review. Neurosci Biobehav Rev 37:1810–1823
-
Kluth K, Steinhilber B, Nesseler T (2013) Oberflächen-Elektromyographie in der Arbeitsmedizin, Arbeitsphysiologie und Arbeitswissenschaft. Z Arbeitswiss 67:113–128
-
Longo L (2018) Experienced mental workload, perception of usability, their interaction and impact on task performance. PLoS ONE 13:e199661
-
Makowski D, Pham T, Lau ZJ, Brammer JC, Lespinasse F, Pham H, Schölzel C, Chen SHA (2021) NeuroKit2: A Python toolbox for neurophysiological signal processing. Behav Res Methods 53:1689–1696
-
Marandi RZ, Madeleine P, Omland Ø, Vuillerme N, Samani A (2018) Eye movement characteristics reflected fatigue development in both young and elderly individuals. Sci Rep 8:13148. https://doi.org/10.1038/s41598-018-31577-1
-
Marquart G, Cabrall C, de Winter J (2015) Review of eye-related measures of drivers' mental workload. Proc Manuf 3:2854–2861
-
Mathôt S (2018) Pupillometry: psychology, physiology, and function. J Cogn 1:1–23. https://doi.org/10.5334/joc.18
-
Matthews G, Reinerman-Jones L, Wohleber R, Lin J, Mercado J, Abich J (2015a) Workload is multidimensional, not unitary: what now? In: Schmorrow DD, Fidopiastis CM (eds) Foundations of augmented cognition. Lecture Notes in Computer Science. Springer, Cham, pp 44–55
-
Matthews G, Reinerman-Jones LE, Barber DJ, Abich J (2015b) The psychometrics of mental workload: multiple measures are sensitive but divergent. Hum Factors 57:125–143
-
McEwen BS, Gianaros PJ (2011) Stress- and Allostasis-induced brain plasticity. Annu Rev Med 62:431–445
-
Mulder LJM (1992) Measurement and analysis methods of heart rate and respiration for use in applied environments. Biol Psychol 34:205–236
-
Myrtek M, Deutschmann-Janicke E, Strohmaier H, Zimmermann W, Lawerenz S, Brügner G, Müller W (1994) Physical, mental, emotional, and subjective workload components in train drivers. Ergonomics 37:1195–1203
-
Nardolillo AM, Baghdadi A, Cavuoto LA (2017) Heart rate variability during a simulated assembly task; influence of age and gender. Proc Hum Factors Ergon Soc Annu Meet 61:1853–1857
-
Oken BS, Salinsky MC, Elsas SM (2006) Vigilance, alertness, or sustained attention: physiological basis and measurement. Clin Neurophysiol 117:1885–1901
-
Parasuraman R (2011) Neuroergonomics: brain, cognition, and performance at work. Curr Dir Psychol Sci 20:181–186
-
Ramsay DS, Woods SC (2014) Clarifying the roles of homeostasis and allostasis in physiological regulation. Psychol Rev 121:225–247
-
Rasmussen J (1983) Skills, rules, and knowledge; signals, signs, and symbols, and other distinctions in human performance models. IEEE Trans Syst Man Cybern 13:257–266
-
Sammito S, Böckelmann I (2016) Factors influencing heart rate variability. Int Cardiovasc Forum J 6:18–22. https://doi.org/10.17987/icfj.v6i0.242
-
Sammito S, Thielmann B, Seibt R, Klussmann A, Weippert M, Böckelmann I (2015) Guideline for the application of heart rate and heart rate variability in occupational medicine and occupational science. ASU Int 2015(06):1–29. https://doi.org/10.17147/ASUI.2015-06-09-03
-
Selye H (1974) Stress without distress, 1st edn. Lippincott, Philadelphia
-
Swan M (2012) Sensor mania! The internet of things, wearable computing, objective metrics, and the quantified self 2.0. J Sens Actuator Netw 1:217–253
-
Van Acker BB, Parmentier DD, Vlerick P, Saldien J (2018) Understanding mental workload: from a clarifying concept analysis toward an implementable framework. Cogn Technol Work 20:351–365
-
Wascher E, Reiser JE, Rinkenauer G, Larrá MF, Dreger F, Schneider D, Karthaus M, Getzmann S, Gutberlet M, Arnau S (2021) Neuroergonomics on the go: An evaluation of the potential of mobile EEG for workplace assessment and design. Hum Factors. https://doi.org/10.1177/00187208211007707
-
Wickens CD (2017) Mental workload: assessment, prediction and consequences. In: Longo L, Leva MC (eds) Human mental workload: models and applications. Springer, Cham, pp 18–29
-
Young MS, Brookhuis KA, Wickens CD, Hancock PA (2015) State of science: mental workload in ergonomics. Ergonomics 58:1–17
griffithbaccumare1950.blogspot.com
Source: https://link.springer.com/article/10.1007/s41449-022-00315-9
0 Response to "Psychophysiological Measures of Workload During Continuous Manual Performance"
Post a Comment